This is the first article about running an AI model on Mac Studio, and I will continue to migrate the environment from CUDA / Nvidia GPU to Mac MPS.
Why did I choose Mac Studio?
I chose Mac Studio because it is less expensive. It has 192GB of memory that can be used as a GPU. This means that it is possible to migrate the program from Nvidia GPU and save some money for personal use.
What is Fuyu-8B?
We are releasing Fuyu-8B, a small version of the multimodal model that powers our product. The model is available on HuggingFace. We think Fuyu-8B is exciting because:
- It has a much simpler architecture and training procedure than other multimodal models, making it easier to understand, scale, and deploy.
- It is designed from the ground up for digital agents, so it can support arbitrary image resolutions, answer questions about graphs and diagrams, answer UI-based questions, and perform fine-grained localization on screen images.
- It is fast – we can get responses for large images in less than 100 milliseconds.
- Despite being optimized for our use case, it performs well at standard image understanding benchmarks such as visual question-answering and natural-image-captioning.
Ok, let’s do it now.
Prepare the environment:
- You need Python 3.6+ and virtualenv installed. Conda or venv also work.
cssCopy code
virtualenv -p python3 py3
- Download the HuggingFace transformers and clone the transformer from GitHub.
bashCopy code
git clone https://github.com/huggingface/transformers.git cd transformers pip install .
- Download the model from https://huggingface.co/adept/fuyu-8b. You can use clone or download it manually, depending on your network speed.
- Install PyTorch from this page https://pytorch.org/get-started/locally/ depending on your environment. Since I am using Mac M2 Studio, I should install this:
Copy code
pip3 install torch torchvision torchaudio
- You are almost done here; now we can start the samples.
Sample 1:
from transformers import FuyuProcessor, FuyuForCausalLM from PIL import Image # load model and processor model_id = "." processor = FuyuProcessor.from_pretrained(model_id) model = FuyuForCausalLM.from_pretrained(model_id, device_map="mps", torch_dtype=torch.float16) # prepare inputs for the model text_prompt = "Generate a coco-style caption.\n" image_path = "bus.png" # https://huggingface.co/adept-hf-collab/fuyu-8b/blob/main/bus.png image = Image.open(image_path) inputs = processor(text=text_prompt, images=image, return_tensors="pt") for k, v in inputs.items(): inputs[k] = v.to("mps") # autoregressively generate text generation_output = model.generate(**inputs, max_new_tokens=7) generation_text = processor.batch_decode(generation_output[:, -7:], skip_special_tokens=True) print(generation_text)
Sample 2:
import os from transformers import FuyuProcessor, FuyuForCausalLM from PIL import Image import torch def list_files_in_directory(path, extensions=[".png", ".jpeg", ".jpg", ".JPG", ".PNG", ".JPEG"]): files = [f for f in os.listdir(path) if os.path.isfile(os.path.join(path, f)) and any(f.endswith(ext) for ext in extensions)] return files def main(): # load model and processor model_id = "." #adept/fuyu-8b" processor = FuyuProcessor.from_pretrained(model_id) model = FuyuForCausalLM.from_pretrained(model_id, device_map="mps", torch_dtype=torch.float16) # To solve OOM, float16 enables operation with only 24GB of VRAM. Alternatively float16 can be replaced with bfloat16 with differences in loading time and inference time. # Load last image path or ask user try: with open("last_path.txt", "r") as f: last_path = f.read().strip() user_input = input(f"Do you want to use the last path '{last_path}'? (yes/no, default yes): ") if not user_input or user_input.lower() != 'no': last_path = last_path else: raise ValueError("User chose to input a new path.") except: last_path = input("Please provide the image directory path: ") with open("last_path.txt", "w") as f: f.write(last_path) while True: # List the first 10 images in the directory images = list_files_in_directory(last_path)[:10] for idx, image in enumerate(images, start=1): print(f"{idx}. {image}") # Allow the user to select an image image_choice = input(f"Choose an image (1-{len(images)}) or enter its name: ") try: idx = int(image_choice) image_path = os.path.join(last_path, images[idx-1]) except ValueError: image_path = os.path.join(last_path, image_choice) try: image = Image.open(image_path) except: print("Cannot open the image. Please check the path and try again.") continue questions = [ "Generate a coco-style caption.", "What color is the object?", "Describe the scene.", "Describe the facial expression of the character.", "Tell me about the story from the image.", "Enter your own question" ] # Asking the user to select a question from list, or select to input one for idx, q in enumerate(questions, start=1): print(f"{idx}. {q}") q_choice = int(input("Choose a question or enter your own: ")) if q_choice <= 5: text_prompt = questions[q_choice-1] + '\n' else: text_prompt = input("Please enter your question: ") + '\n' while True: # To enable the user to ask further question about an image inputs = processor(text=text_prompt, images=image, return_tensors="pt") for k, v in inputs.items(): inputs[k] = v.to("mps") # To eliminate attention_mask warning inputs["attention_mask"] = torch.ones(inputs["input_ids"].shape, device="mps") generation_output = model.generate(**inputs, max_new_tokens=50, pad_token_id=model.config.eos_token_id) generation_text = processor.batch_decode(generation_output[:, -50:], skip_special_tokens=True) print("Answer:", generation_text[0]) text_prompt = input("Ask another question about the same image or type '/exit' to exit: ") + '\n' if text_prompt.strip() == '/exit': break #if name == "main": main()
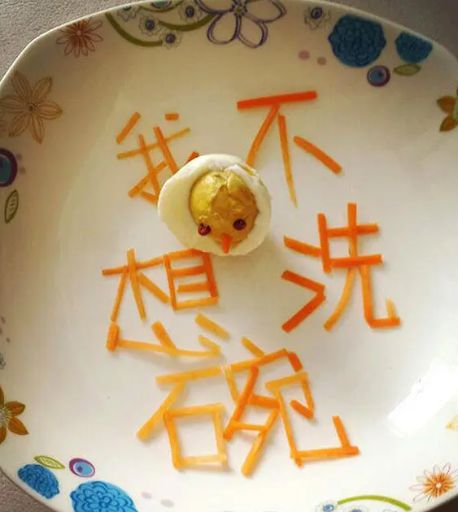

yes, It is Chinese. But not the Chinese fuyu-7b knows. It is not “食” (eating) , but “我不想洗碗”( i don’t want to wash the dishes). Fuyu-7b is lying. lol.